Bridging the gap between data and action
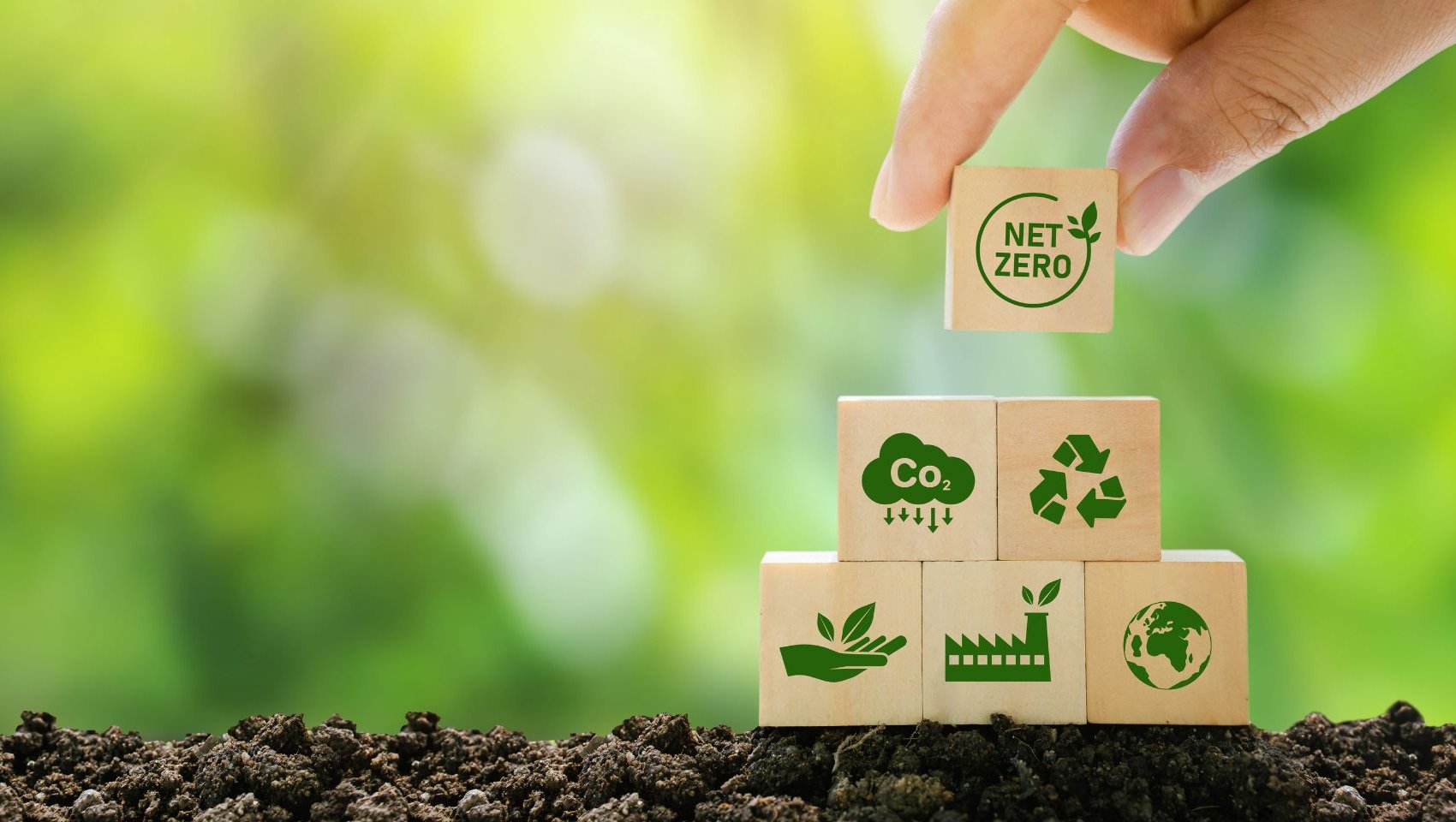
Corporate sustainability data is becoming increasingly widespread and thorough. Emerging sustainability regulations, such as the Corporate Sustainability Reporting Directive (CSRD), are putting more obligations on companies to report on sustainability and we are seeing great advances in the field. Yet there is a difference between data and action.
More than one-third of the world’s largest publicly traded companies now have net zero targets. However, according to Net Zero Tracker, two-thirds of these companies haven't publicly made it clear how they plan to achieve that goal. To get to net zero, companies need to establish clear pathways based on predicted sustainability outcomes.
Over the past two years at ESMT Berlin, we have developed action-oriented and research-based insights which support companies on a path from net zero targets to net zero business. These include the following recommendations and strategies.
Maximize an abundance of internal data
Contrary to the popular opinion that sustainability data is difficult to obtain, we have observed an abundance of sustainability relevant data available within companies. Very often, internal stakeholders from sustainability departments lack a good overview of what data on environmental, social, and governance factors is collected across the company, in different business departments. For example, a wide array of social sustainability indicators is often tracked by HR departments. This data, if a proper channel and process for access are established, can be used for improving sustainability performance.
The starting point is conducting an internal assessment of relevant data available across different functions. In the next step, gaps, inconsistencies, infrastructural challenges, and required enhancements in data format and coverage need to be addressed. This could include, for instance, tracking the number of requests for sustainable products from customers in order to steer the product portfolio. Similarly, it would be impactful to track investments on environmental reduction technologies and estimate their corresponding reduction in CO2.
Monitor external developments and trends
Companies need to track external sustainability trends, such as sustainability regulation, environmental innovation, public sentiment, as well as the sustainability performance of competitors, suppliers, and customers.
These measures and external data sources helped create evidence that there is indeed an increased and universal pressure on companies to become more sustainable. This was indicated by the increasing number of environmental technology patents, more sustainability regulations, improving environmental performance from industry peers, and stronger social pressure to operate sustainably.
Interestingly, we established that these trends are not, contrary to the popular feeling in our location, only present in the EU but also, for instance, in China and Korea.
Use economic modelling for scenario planning
The FUTURE Institute for Sustainable Transformation at ESMT Berlin, in collaboration with Merck KGaA, Darmstadt, Germany, a leading science and technology company, have developed a modelling framework shedding light on societal trends around climate change and modelled their impact on corporate emissions.
The central contribution of our project has been a predictive model for climate-related outcomes, yielding several scenarios for greenhouse gas (GHG) emissions-related societal trends.
Predictive modelling is not new. In economics and other fields, decisions are now routinely driven by modelling outcomes based on historical data. But little rigorous modelling of sustainability outcomes is available for analysis. That’s because this strategy just hasn’t been broadly applied to sustainability, yet.
Economic forecasting typically uses data points that span large time horizons with relatively high time frequency. For sustainability forecasting, we do not have this luxury. Sustainability data has only been collected in recent times, and the further back in time we go, the less reliable the data quality becomes. So, to reach an acceptable model performance, we needed to think creatively. We decided to feed the model not just internal climate-related data, but also data from sources that might seem at first to be only indirectly linked.
For testing, these factors were added to a rich modelling framework, using advanced analytical methods. This led to a predictive model for Merck KGaA, Darmstadt, Germany’s direct (scope 1) and indirect (scope 2) greenhouse gas (GHG) emissions. With this model, the company was able to generate predictive outcomes for a mid- and long-term horizon under a range of scenarios considering multiple variations of the modelling factors.
The overall range of predictive outcomes offers the potential to create a more holistic understanding of climate-related impacts. We recommend other companies make use of internal and external data as well as economic modelling tools to better understand how and when they can achieve their net zero targets.
ESG data challenges to solve next
In this collaborative research process, we tackled several data and modelling challenges. First, in order to obtain reliable and sufficient information on all the external factors, we needed to mine large amounts of data from a range of sources. Advanced analytics were key for this task.
The second challenge had to do with the data itself. A lot of sustainability data is gathered by various organizations, using many data platforms and providers. But for any practical application, all this data is relatively unstandardized. There is no clear, standard and generally accepted solution for how to measure various outcomes. Even those outcomes that are measurable may be subject to measurement errors. Much data is missing. Commercial data platforms providing sustainability data often provide information that proves inconsistent when being compared across sources.
Finally, the present study focuses only on scope 1 and scope 2 emissions – these are emissions resulting directly from a company’s operations or from generating the energy that a company purchases. Building on this, it would be worthwhile looking into modelling and predicting scope 3 emissions. These occur in the value chain of a company, but are not owned by the company itself. They are generated in upstream activities, such as distribution, travel not using company vehicles, and purchase of goods and services, as well as in downstream activities, such as investment and the use of sold products.
In this next step, the modelling challenges will be greater, because the existing data on scope 3 emissions is very limited. Moreover, the current estimation methods lead to much less reliable measurements than data on scope 1 and 2 emissions.
However, the range of available analytical tools and the actionable insights from the success of the project between the FUTURE Institute and Merck, KGaA, Darmstadt, Germany give us confidence that creative approaches for the deployment of these analytical solutions to sustainability data can be found and will lead to novel predictive frameworks. Thus, analytics can, once again, provide tremendous additional business value by assisting companies to make sustainable strategic decisions.
This article was originally published in The European | Climate Change Review, Issue 08.