AI and its importance for the startup ecosystem – a European perspective
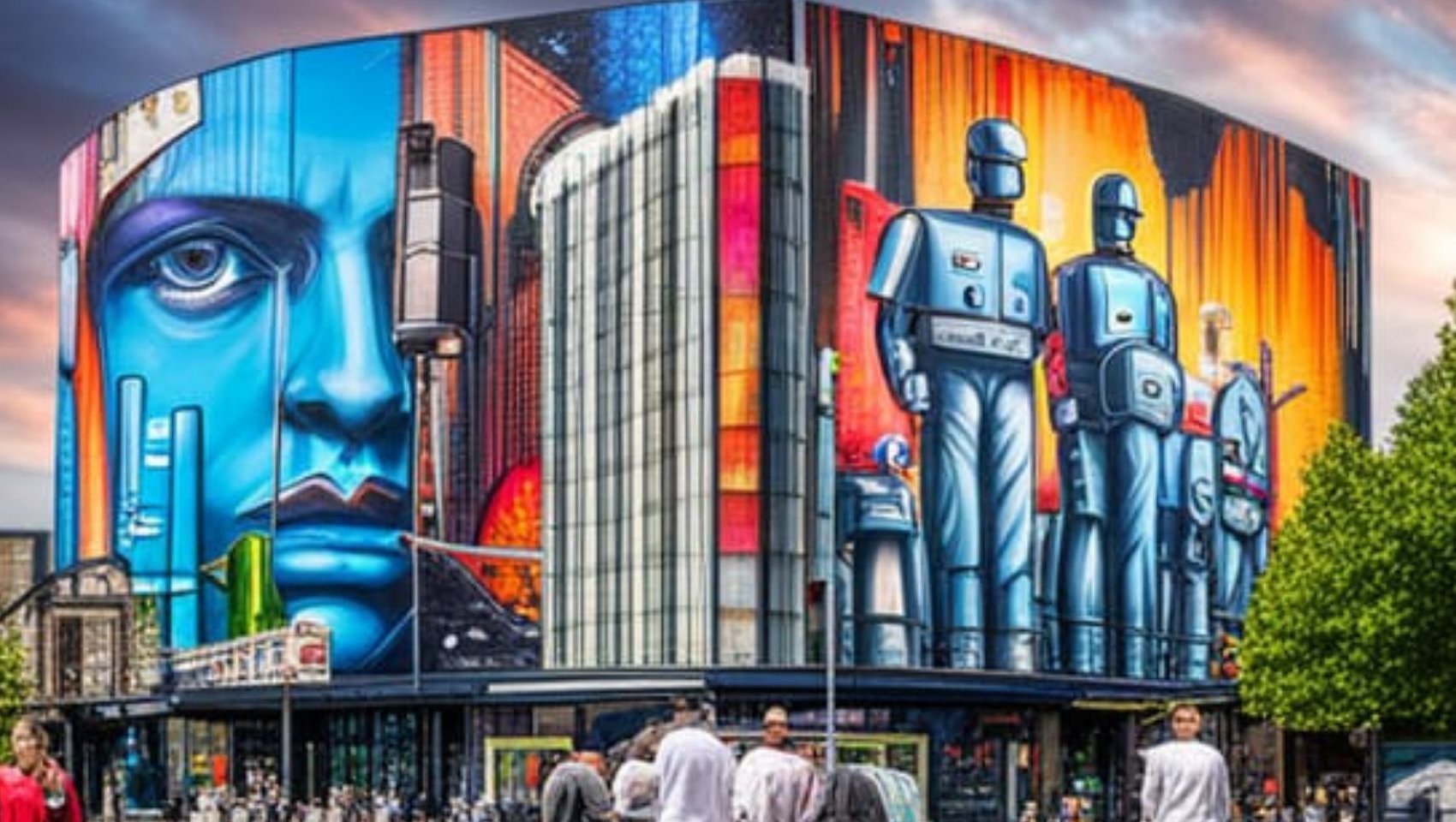
This article analyzes the significance of AI foundation models for Europe’s AI startup ecosystem and highlights the possibility that Europe might once again miss its chance to achieve digital sovereignty. Will Europe’s AI capabilities catch up or fall behind?
AI has gained increasing prominence in recent years, driven by advancements in machine learning, natural language processing, as well as an increasing availability of processing power, and data. The launch of OpenAI’s ChatGPT in late 2022 demonstrated AI’s disruptive potential. It is the fastest app to reach 1 million users, achieving this feat in just five days and reaching 100 million users in just two months.
Even though ChatGPT has garnered unprecedented attention for AI within society, the technology has been used in the startup ecosystem for quite some time. B2B software-as-a-service (SaaS) startups already leverage AI to optimize processes, enhance predictions, and automate decisions in various industries and functions, including healthcare, autonomous driving, and supply chain. AI startups and their underlying models are expected to have a transformative impact, with generative AI startups attracting $10 billion and Microsoft investing another $10 billion in OpenAI since its launch.
The challenge that all companies must confront today is determining which decisions are left to AI, which to humans, and which require collaboration. Moreover, it will be important to identify which capabilities of both AI systems and humans will be employed to increase value creation, while also considering societal consequences of technological progress. Regardless of the specific answer to this question, building AI-related capabilities and infrastructure will help AI startups and their ecosystems to flourish.
What model is behind ChatGPT and why will it be so important for Europe to build its own one?
ChatGPT and foundation models
ChatGPT initial launch was based on the large language model GPT-3, an abbreviation for Generative Pre-Trained Transformer 3. GPT-3 is trained on vast amounts of data and uses enormous processing power to generate human-like responses. It has already a deep understanding of almost all human knowledge and language to predict the next word in a passage. However, the strength of ChatGPT lies in incorporating human feedback after its initial pre-training. This deep reinforcement learning was the key to creating responses that became astonishingly real or – perhaps – “human.”
Just six months later, OpenAI launched a new version of its underlying foundation model, GPT-4, which is trained on 500 times more parameters and is multimod.al. Its text and image multimodality is a technological breakthrough and enables new interactions with the AI.
What are the implications of foundation models for startups?
The foundation models behind ChatGPT serve as a catalyst for novel AI applications by startups. Instead of building algorithms and datasets from scratch, startups can connect via an API to these foundation models and build on functionalities, such as reasoning or code generation. This leads to a massive reduction in the costs of implementing AI and establishing an AI company, thus accelerating the creation of new AI use cases and companies. For example, Microsoft implemented OpenAI into various applications, including search (Bing) and Microsoft Office (Excel, Word, etc.). Moreover, non-technical founders may build a business model around basic AI without having to develop AI systems themselves, for example, a chatbot for salespeople or drug classification in pharma. An increasing number of resources are flowing into developing new business models, leading to faster progress of the technology and adoption of AI into society. On the other hand, foundation models reduce entry barriers, thus increasing the competition in the AI startup ecosystem. If foundation models become exponentially better, it will make it more difficult for startups to differentiate and build a competitive advantage.
Challenges of developing European AI capabilities
LEAM, an initiative of the German AI Association and leading representatives from industry and research, analyzed the importance of foundation models for the digital sovereignty of Europe. Its recent research shows that 73 percent of foundation models are developed in the US and 15 percent in China. Despite Europe’s significant scientific progress in AI, several challenges hinder the region’s ability to compete with the US and China. According to LEAM, key challenges include:
Insufficient tech transfer and retention of AI talent
European universities educate some of the best AI experts in the world. However, many of these talents work afterward in the US or for US companies. In most cases, development opportunities, resources, and salaries in the US are much better than in Europe. The German AI Association estimates that approximately €350 to €400 million are needed in public and private investment for an AI research center. Moreover, while Europe has made significant scientific progress in AI, it lacks in commercializing and transferring its IP into the economy. There is no easy answer to this question. However, easier IP transfer as well as a joint AI research center would likely improve the scientific progress, its commercialization, and retention of post-graduate AI talent in the EU.
Data, processing power, and computational infrastructure
Collecting large data sets will remain a tremendous challenge for European startups. More than 50 percent of startups consider data protection laws an international competitive disadvantage. Similarly, processing power is important for an algorithm’s effectiveness, which requires expensive computational infrastructure. Furthermore, Germany is famous for
its Mittelstand, which has skilled engineers but often lags in digitalization efforts. Many still use Excel and poorly document data, which cannot be used for AI applications easily. For European AI startups to flourish, we need to invest heavily in computational infrastructure and, thus, enable processing power needed to train AI algorithms and better use data.
Lack of funding and dependence on foreign capital
Even though the European VC industry – especially in generative AI – is emerging, there is still significant dependence on foreign investors and a massive lack of funding compared to the US. Around 42 percent of the money that went into startups came from Asian or American investors. Hence, to enable startups to invest in technology – for example, to hire scientists or finance processing power – we need much more European funding to invest in the development of LLMs and fund the next generation of AI startups.
Missing big players with “AI-first” strategies
Currently, most foundation models are developed by businesses rather than academic labs, as training these algorithms requires enormous investment in hiring scientists and engineers, building expensive AI computational infrastructure, and financing the processing power. In addition to Microsoft’s investment in OpenAI, other Big Tech companies are developing foundation models, too. For example, Google announced its foundation model, Bard, in early 2023. In Europe, we need large players with AI-first strategies. With the resources and the ability to take great risk to develop, train, and improve foundation models, they are crucial for the development of AI and AI capabilities. And, of course, they have massive data sets that are needed to give AI its predictive power.
Changing the European regulatory landscape
To address the aforementioned challenges, the EU is planning to launch the AI Act. This act is a set of regulations designed to ensure that AI technologies are developed and used in a safe, transparent, and ethical way. The act’s key provisions include:
• a ban on certain types of AI technologies that are high risk, such as those that are used for mass surveillance or social scoring
• requirements for transparency in AI systems, so users understand how systems make decisions
• rules for data protection and privacy to ensure that personal data is not misused or abused
• guidelines for AI developers and users to help them understand how to comply with regulations
The EU AI Act seeks to regulate AI and control its risks, potentially affecting the ability of startups to innovate. While the act has been praised for its focus on ethical and transparent AI development, some critics argue it may disproportionately benefit large corporations, which can more easily fulfill compliance requirements. A study by the state of Bavaria and applied AI estimates that up to 50 percent of AI algorithms might be classified as high risk, which leads to high compliance requirements for AI startups.
In contrast to startups, large tech companies like Mi.crosoft and Google have enough money to afford to meet regulatory requirements. For example, Aleph Alpha is a promising deep-tech AI company from Germany that develops an AI foundation model called Luminous. Even though it has only 1 percent of the funding of OpenAI, a recent test shows that its model is almost as good as the models from OpenAI, Meta, and others. Nevertheless, in addition to a lack of funding, Aleph Alpha still suffers from the mentioned challenges including processing power, computational infrastructure, data, and retention of AI talent.
Conclusion
Humans usually overestimate how fast a disruptive technology affects society. However, probably no technology has spread over the world as fast as AI. As AI develops into a general-purpose technology, it opens a new world of value creation and opportunities, but also risks for our society and economy. Foundation models accelerate the adoption of AI, becoming a platform for AI startups to emerge, identify use cases, and build business models faster. Hence, to ensure that European AI startups have no competitive disadvantage, it will be crucial to develop AI foundation models. The existence of companies such as Aleph Alpha show that it is possible. However, the aforementioned challenges – such as lack of funding, poor retention of talent, and the lack of computational infrastructure, data, and processing power – will make it even more difficult in the long run.
In a global context, leveraging AI and its opportunities, while mitigating risks, will require bold policy action as well as collaboration between business leaders, startups, technologists, and other relevant stakeholders, such as policymakers. To find the right balance between the two will become the major challenge to build up European AI capabilities and ensure future digital sovereignty in Europe.